Comparison of Invoice OCR Systems in the Age of Generative AI
Last update:
15.10.2024
5 minutes
🔍 Looking for the best way to analyze your invoices in detail? Every line of your invoice contains vital data for your business: expense analysis, verification of pricing accuracy, and tracking price variations by item. Extracting this information can be complex; OCR (Optical Character Recognition) tools are essential for this process, but which one is the most suitable?
The line-by-line details of invoices, that is, the specifics of each good and service billed, hold vast amounts of granular data about your purchases. However, today this data often remains underutilized, as it is frequently restructured and varies by supplier. Yet, companies can unlock decisive advantages through detailed line items:
- In accounting, to ensure the correct processing of each invoice.
- In management control**, to verify compliance with the pricing grid or detect fraud.
- In procurement management**, to track price variations and have the data to negotiate with suppliers and compare the price paid for each reference.
The challenge is to convert this unstructured billing data into a structured database for easy information exploitation and analysis.
Software relying on OCR technology is the first building block to access this data. Most software today achieves very satisfactory results for extracting general information such as supplier name, total amount excluding and including tax, addresses, and VAT numbers.
However, the crucial line-by-line detail still lags behind. We tested different OCR software on two sets of invoices to understand their performance:
- 15 "easy" invoices that presented no particular difficulties, in native PDF format, with clean line-by-line detail in a clearly defined table, without extraneous information.
- 15 "difficult" invoices that included challenges such as scanned PDFs, descriptions spanning multiple lines or truncated over several pages, and extraneous information like total lines and non-standard fields.
To achieve the goal of structuring billing data, OCR must excel in two areas:
- Success rate: This is the ability to recognize and extract the various fields from the line items. This rate should be close to 100%—a slightly lower rate, such as 95%, renders the data unusable, as it needs verification.
- Configurability: This is essential to recognize fields that are truly relevant for the use case (e.g., SKUs, EANs, business codes, the relevant period, quantity units, etc.) and to create a uniform database despite the diversity of invoices received.
Why Koncile?
To recognize line-by-line details, Koncile combines visual recognition technologies to detect the organization of invoices with generative AI to enhance understanding. To perfectly adapt to your business environment, Koncile’s OCR allows for dynamic customization of certain fields to extract and provide instructions.
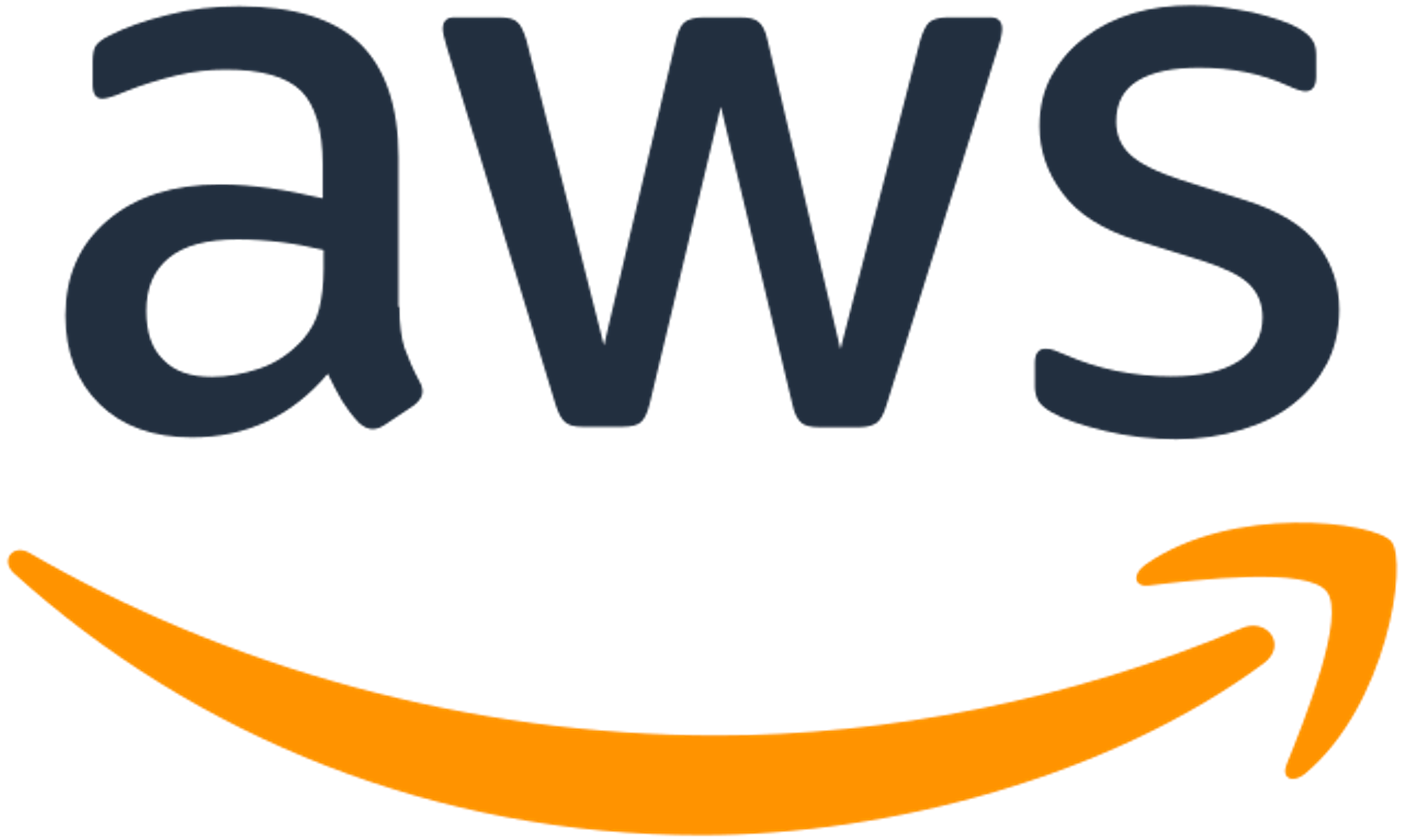
Amazon Textract Analyse Expense
Recognition of Main Fields. The AWS tool can recognize 43 fields from invoices, including key elements such as names, addresses, total amounts excluding and including tax, and even some more specific predefined fields like shipping costs or payment terms. The success rate for these main fields is close to 100% when they are present on the invoice.
Line-by-Line Recognition ⭐⭐✨✨✨ (2/5). The tool offers a line item fields section that recognizes the line-by-line details of invoices. For "simple" invoices, the line item information is accurately extracted into an Excel table for 14 of them. The spreadsheet is specific to the fields present in each invoice. However, among the 15 "complex" invoices, more than 10 had significant errors: missing lines, missing descriptions, or the addition of irrelevant lines. The difficulty arises from the fact that field recognition is primarily based on computer vision rather than linguistic understanding. It is advisable to use the tool on invoices with a simple organization in native PDF format, rather than scanned PDFs.
Configurability ⭐⭐⭐✨✨ (3/5). The tool does not allow for the extraction of specific fields from the invoice, such as a number unique to your business environment. You need to use another feature, AnalyzeDocument - Queries, to formulate specific extraction requests. Similarly, if you have multiple suppliers with different types of invoices to extract, the tool does not allow you to obtain a consolidated Excel file with the same fields extracted from the line items, making data analysis more challenging.
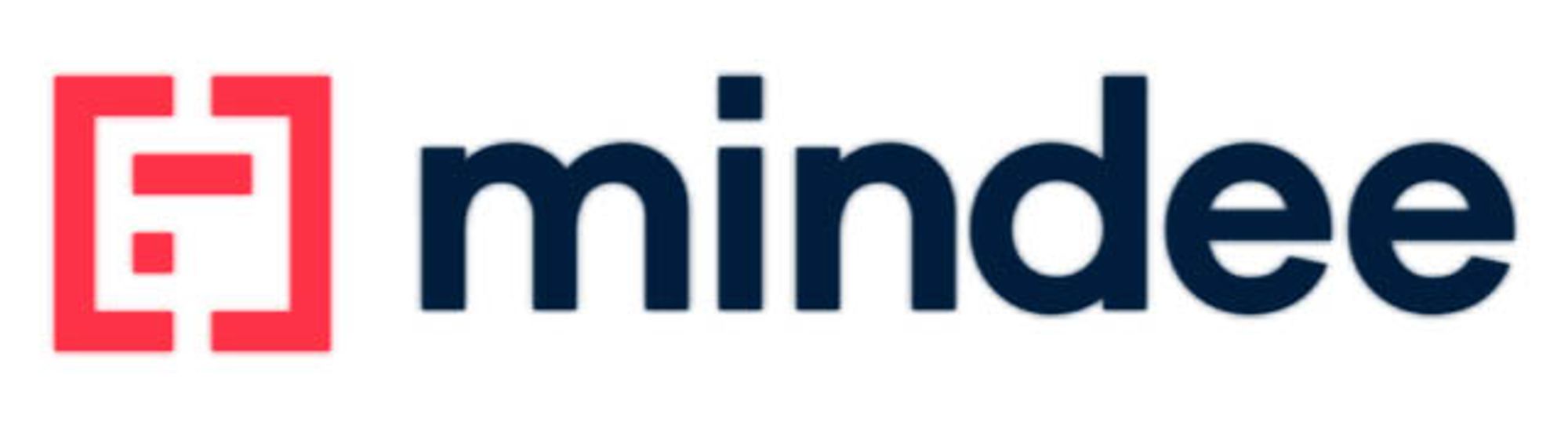
Mindee Invoice parser
Mindee offers an off-the-shelf invoice OCR that detects 16 main fields. In the tested panel, the success rate for this basic information is close to 100%, particularly for scanned invoices.
Line-by-Line Recognition ⭐⭐⭐✨✨ (3/5). Mindee provides a list of "default" fields to extract line-by-line: description, product code, quantity, unit price, total price, and VAT. For 9 out of the 15 "complex" invoices, errors were detected whenever the table formats were less standardized. Key information is sometimes overlooked: for example, a product code may be recognized instead of a SKU or an EAN code. Using this data will still require significant post-processing in Excel and verification of the information.
Configurability ⭐⭐⭐✨✨ (3/5). Mindee allows for the extraction of specific information through its API Builder module. You will need to "train" the tool to extract the desired information by annotating a few dozen identical documents. It is not possible to simply "ask" in natural language form to obtain the result.
Speed and Ease of Use ⭐⭐⭐⭐⭐ (5/5). For the 30 invoices tested, the average time per page is about 5 seconds.
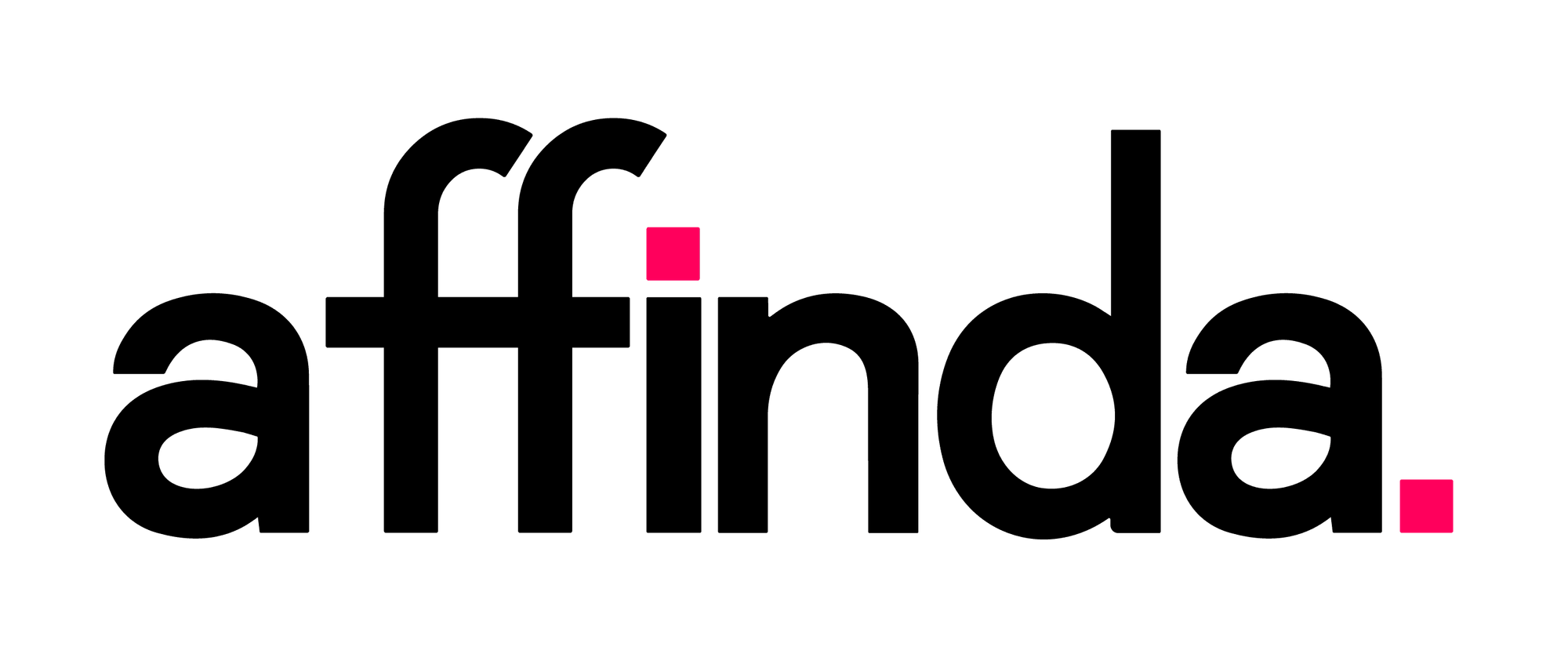
Affinda
Affinda's tool offers a set of general fields to extract by default from invoices. Of the 30 invoices tested, 5 contained errors in at least one key field, such as the customer's SIRET number or the total invoice amount.
Line-by-Line Recognition ⭐⭐✨✨✨ (2/5). Affinda provides a line-by-line detection system using a table detection method. Out of the 15 "complex" invoices, 7 yielded usable results. However, when descriptions exceed multiple lines, many extraneous lines are created, making the information non-standardized and difficult to utilize.
Configurability ⭐⭐⭐⭐⭐ (5/5). The tool allows you to configure the fields to be extracted, add new ones, or remove existing ones based on a large language model (GPT). However, it is not possible to configure the extraction of line items.
Speed and Ease of Use ⭐⭐⭐⭐⭐ (5/5). The tool includes a feature for correcting erroneous information and has the capability to learn from the company’s data (not tested).
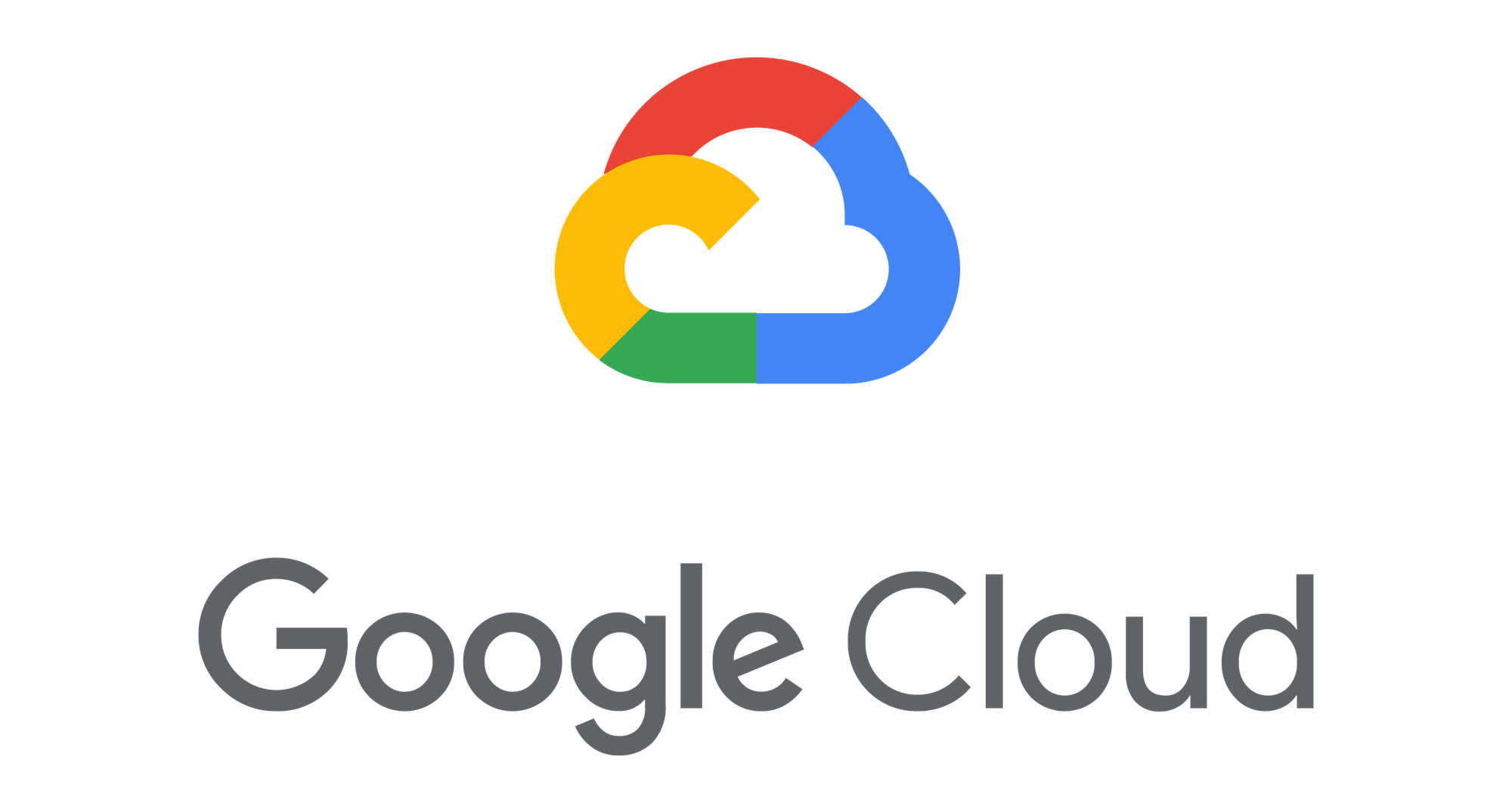
Google Document AI
Recognition of Main Fields ⭐⭐⭐⭐⭐ (5/5). The Invoice Parser tool offers 37 fields to extract from invoices via the Document AI console. These fields are not modifiable or editable.
Line-by-Line Recognition ⭐⭐⭐✨✨ (3/5). The tool extracts a fixed list of 7 line item details: quantity, description, product code, purchase order, number, unit, and unit price. These fields are fixed, which does not allow for adaptation to specific business information or handling multiple codes. While the success rate is high for "simple" invoices, many key details for complex invoices are not extracted, and entire lines are sometimes overlooked.
Configurability ⭐⭐✨✨✨ (2/5). Document AI allows you to create a dataset of invoices and train it to recognize certain information (not tested).

Nanonets
Recognition of Main Fields ⭐⭐⭐⭐✨ (4/5). Nanonets is a dedicated document OCR solution that includes invoices in its range of processed documents. It extracts 28 fields by default and allows you to customize extraction formats for each field (date, currency, etc.).
Line-by-Line Recognition ⭐⭐✨✨✨ (2/5). Nanonets extracts line-by-line information based on table recognition, similar to Affinda's approach. Of the 15 "complex" invoices, some columns are sometimes excluded from recognition, often concerning key data such as product codes or unit prices.
Configurability ⭐⭐⭐✨✨ (3/5). The pro version allows you to create training datasets to specify where information is located. This feature is relevant for long documents but is rather challenging to apply to the line items of invoices.
Ease of Use ⭐⭐⭐⭐⭐ (5/5). Nanonets offers integrations with Google Drive, easy export to Excel, and invoice approval workflows.

Parsio
The PDF-parser tool (pre-trained model) provides a fixed number of fields to extract from invoices. For these general fields (excluding line items), it achieves extraction results with an accuracy close to 100% for "easy" invoices and 97% for "complex" invoices.
Line-by-Line Recognition ⭐⭐⭐✨✨ (3/5). For the 15 complex invoices, line-by-line extraction is accurate for 10 of them. However, challenges remain for non-scanned PDFs. Since line-item configuration is not possible, one number may be mistaken for another, and users cannot correct errors or train the machine to find the correct element. Thus, it is difficult to create a uniform pricing database with the extracted data.
Configurability ⭐⭐⭐✨✨ (3/5). Parsio offers a feature to search for fields by prompt based on GPT-4. This allows for the extraction of specific data from documents. However, this functionality cannot be used for line-item recognition, making it impossible to identify relevant fields across all services and goods billed. Additionally, it is not yet combined with OCR, so it can only read source PDFs and does not account for page organization.
Ease of Use. The web app generates an email address to which documents can be sent. A wide range of integrations is possible.
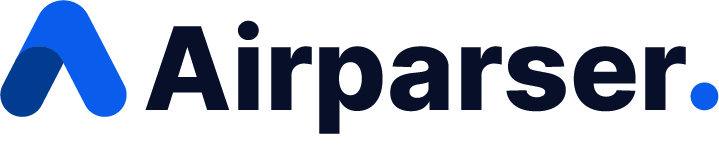
Airparser
The tool leverages GPT-4 technology to extract specific fields from any type of document. It is built by the same publisher as Parsio.
Line-by-Line Recognition and Configurability ⭐⭐⭐✨✨ (4/5)
The tool allows you to configure the fields you want to extract. With the "list and table" function, you can extract billing lines by defining the various attributes of each line. For each field, you add a description that helps the tool refine extraction accuracy. "Simple" invoices yield satisfactory results when attribute descriptions are sufficiently precise. However, for complex invoices, we noted confusion between columns. The risk of error is notably higher in the presence of scanned invoices.

Base64.ai
Base64 offers an off-the-shelf invoice extraction tool, systematically extracting a set of fields.
Line-by-Line Recognition ⭐⭐⭐✨✨ (3/5). 14 out of the 15 "simple" invoices are extracted with a good success rate. For complex invoices, issues with multiple numbers, page breaks, or information contained in headers are not addressed for 5 invoices.
Configurability ⭐⭐⭐✨✨ (3/5). The tool allows you to ask a question about the document or add an extracted field. However, it does not allow for modifying the extracted fields in each line or providing specific instructions.
Ease of Use. Response time can be up to a minute for long invoices. Many integrations are planned in document management "flows."
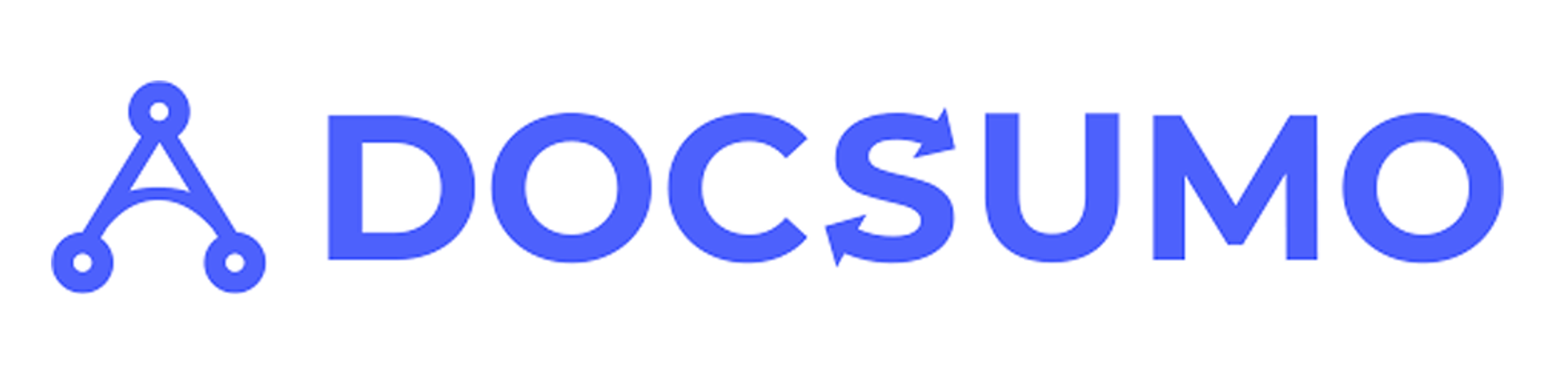
Docsumo
Docsumo offers an off-the-shelf tool that extracts the main fields from invoices.
Line-by-Line Recognition ⭐⭐✨✨✨ (2/5). The tool extracts line-by-line information using table detection, similar to the OCR of Nanonets or Affinda. This works well when all information relating to a line is well-aligned. However, for complex tables, it is not possible to capture relevant information.
Configurability ⭐⭐✨✨✨ (2/5). A "ChatAI" feature allows you to ask questions about the document. However, responses cannot currently be systematically integrated into the extracted fields. The tool does not provide a function to specify or modify the various extracted fields or the line items.
Try Koncile today
C
Resources
T
This article presents methods currently used to extract tables from scanned documents.
Practical guide
F
Article presenting a list of 8 interesting features to have in your ERP if you work in the construction sector.
Practical guide
&
With technology, automate the checks of your supplier invoices.
Practical guide